Keynote Speakers
Prof. George Q. Huang
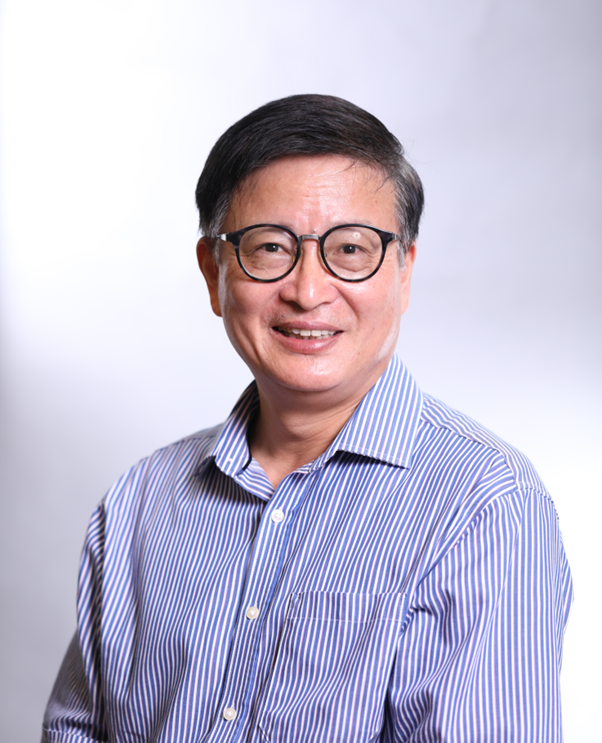
Prof. George Q. Huang, Chair Professor of Smart Manufacturing, Department of Industrial and Systems Engineering, The Hong Kong Polytechnic University, Hong Kong SAR
Bio: George is Chair Professor of Smart Manufacturing at Department of Industrial and Systems Engineering, The Hong Kong Polytechnic University. He gained BEng and PhD in Mechanical Engineering from Southeast University (China) and Cardiff University (UK) respectively. He has conducted research projects in areas of Smart Manufacturing, Logistics, and Construction Systems Analytics through IoT-enabled Cyber-Physical Internet with substantial government and industrial grants exceeding HK$120M. He collaborated closely with industries through joint projects and start-up companies. He has published extensively and his works have been widely cited by research communities. He serves as associate editors and editorial members for several international journals. He is Chartered Engineer (CEng), Fellow of ASME, CILT, HKIE, IET, and IISE.
Title of Keynote: Cyber-Physical Internet (CPI): Next-generation of resilient logistics of manufactured products
Abstract of Keynote: The talk is about a major research project on Cyber-Physical Internet (CPI) recently initiated in Hong Kong. Its vision is to establish a new paradigm for sending and receiving manufactured goods just like sending and receiving instant messages over the internet using online chatting platforms. Four innovations are critical to achieve this ultimate vision: (1) digitization architecture for entangling the flows of information and materials into one flow of cyber-physical objects for manufacturing and logistics operations; (2) network services for configuring local aera network (LAN), wide area network (WAN) and catchment area network (CAN); (3) value mechanisms to motivate and facilitate participation and collaboration between multiple stakeholders including shippers, carriers, forwarders; and (4) decision analytics for synchronized logistics planning, scheduling and execution. These innovations are based upon some fundamental breakthroughs of CPI routers and TCP/PIP (Transmission Control Protocol / Physical Internet Protocol) protocols that are yet to be developed. CPI contributes to establishing post-pandemic “new norms”, while logistics resilience and CO2 emission targets are achieved.
Prof. Barbara Hammer

Prof. Barbara Hammer, Full Professor for Machine Learning at the CITEC Cluster at Bielefeld University, Germany.
Bio: Barbara Hammer is a Full Professor for Machine Learning at the CITEC Cluster at Bielefeld University, Germany. She received her Ph.D. in Computer Science in 1999 and her venia legendi (permission to teach) in 2003, both from the University of Osnabrueck, Germany, where she was head of an independent research group on the topic ‘Learning with Neural Methods on Structured Data’. In 2004, she accepted an offer for a professorship at Clausthal University of Technology, Germany, before moving to Bielefeld in 2010. Barbara’s research interests cover theory and algorithms in machine learning and neural networks and their application for technical systems and the life sciences, including explainability, learning with drift, nonlinear dimensionality reduction, recursive models, and learning with non-standard data. Barbara has been chairing the IEEE CIS Technical Committee on Data Mining and Big Data Analytics, the IEEE CIS Technical Committee on Neural Networks, and the IEEE CIS Distinguished Lecturer Committee. She has been elected as member of the IEEE CIS Administrative Committee and the INNS Board. She has been an associate editor of the IEEE Computational Intelligence Magazine, the IEEE TNNLS, and IEEE TPAMI. Currently, she is involved in a number of large-scale projects including the DFG collaborative research center on Constructing Explainability, the EU Doctoral Network on Learning with Multiple Representations (LEMUR) and the ERC Synergy Grant Smart Water Futures.
Title of Keynote: Challenges of Trustworthy AI
Abstract of Keynote: In recent years, AI technologies have led to breathtaking successes in diverse areas including computer vision, natural language processing, or insights into biology and medicine. This way, computers reach human or super-human capabilities in many areas. Yet at the same time, machine learning methods face possibly harmful errors which are at odds with human expectations. Adversarial examples in computer vision tasks constitute one prominent example for a misalignment of computer vision and human visual perception. Other examples of misalignment are AI-based decision models which systematically discriminate persons based on ethnicity or gender, or machine learning language models which hallucinate wrong facts. Within the talk, I will address two modeling paradigms which constitute a step towards mitigating such effects: (1) Incremental learning from data streams: One cause for AI failures is given by the assumption that models act in a stationary environment. In practice, the underlying setup changes due to e.g. ageing sensors, changed demands of costumers, seasonal effects, etc. Hence reliable models need to detect such changes and adjust a model accordingly. In the first part of the talk, I will address the challenge of incremental learning from streaming data which might be subject to drift. I will present how to efficiently learn in such setups, and how to detect and explain drift in such scenarios. (II) Explainability: Another cause for AI failures is caused by a misalignment of human’s objective and its mathematical formalization within an AI model. One way to uncover such misalignments is by explanations. In the talk, I will address counterfactual explanations as one particularly intuitive form of local explanation. I will deal with the question how to efficiently compute those and how to use them in modelling pipelines. In addition, I will bridge incremental learning and explanations by having a glimpse at explanations of drift.
Prof. Dawn Tilbury

Prof. Dawn M. Tilbury, Ronald D. and Regina C. McNeil Department Chair of Robotics and the Herrick Professor of Engineering at the University of Michigan.
Bio: Dawn M. Tilbury is the inaugural Ronald D. and Regina C. McNeil Department Chair of Robotics at the University of Michigan, and the Herrick Professor of Engineering. She received the B.S. degree in Electrical Engineering from the University of Minnesota, and the M.S. and Ph.D. degrees in Electrical Engineering and Computer Sciences from the University of California, Berkeley. Her research interests lie broadly in the area of control systems, including applications to robotics and manufacturing systems. From 2017 to 2021, she was the Assistant Director for Engineering at the National Science Foundation, where she oversaw a federal budget of nearly $1 billion annually, while maintaining her position at the University of Michigan. She has published more than 200 articles in refereed journals and conference proceedings. She is a Fellow of IEEE, a Fellow of ASME, and a Life Member of SWE.
Title of Keynote: Digital Twins for Manufacturing Systems: Improving Productivity and Expanding Capabilities
Abstract of Keynote: Digital Twins have the potential to reduce cost, improve quality, and expand capabilities in manufacturing systems. As computing and networking technologies improve, the massive amounts of data being collected on manufacturing plant floors can be leveraged through digital twins to create useful information and advise human operators on recommended actions. Even with the huge amount of data available, data quality remains an important challenge. Standards for Digital Twins are emerging, and there are opportunities to create different types of Digital Twins that can best utilize the data that exits. In this talk, we will present a requirements framework for Digital Twins in the manufacturing domain, including the important properties of re-usability, interoperability, interchangeability, extensibility and maintainability. Several examples of digital twins that we have created, in collaboration with our industry partners, will be presented, covering multiple application domains. Future challenges and opportunities in the area will also be discussed, including the automation of the development and maintenance of digital twins.
Prof. Mike Duke

Prof. Mike Duke, Dean of Engineering and Dr John Gallagher Chair in Engineering at the University of Waikato, New Zealand.
Bio: Professor Mike Duke is the Dean of Engineering and the Dr John Gallagher Chair in Engineering at the University of Waikato. He is a founding member of the Waikato Robotics Automation and Sensing (WaiRAS) research group. Mike’s group works with growers and robot manufacturers undertaking research into automating horticultural processes. His research includes human assist technologies as an intermediate step towards full automation, electric and hybrid autonomous orchard vehicles, specialist robotic hardware for horticultural tasks and smart automation to replace obsolete horticultural machinery. He was the lead academic on the hardware development of the MBIE Horticultural Robotics project and leads the hardware research on the MBIE MaaraTech: Data informed decision making and automation in orchards and vineyards.
Title of Keynote: Horticultural Robotics – Challenges and Opportunities, a New Zealand Perspective
Abstract of Keynote: Horticultural produce is one of New Zealand’s main exports and labour shortages are an on-going threat to the industry. Many other countries face a similar labour problem with regular news stories of valuable crops rotting in fields. Robotics is perceived by many as the way to solve the labour shortage and globally a wide range of prototypes and early market robotic products have been developed. However, nearly all of them face challenges to be commercially viable. These challenges are explained, and as an early developer of horticultural robotics, examples from New Zealand will be used to demonstrate how we have started overcoming these challenges. We will also present the important factors for a successful horticultural robotics industry, including; a ‘systems’ approach for the entire crop cycle, co-design methods for developing viable solutions, safety challenges for autonomous orchard vehicles, the value of ‘human assist’ technologies as an intermediate step towards full automation, the importance of high quality validated data for accurate ‘in orchard’ decision making, developing growing structures that compliment automation and how computer vision and machine learning, combined with best practice horticultural process algorithms can help automate critical tasks such as pruning.